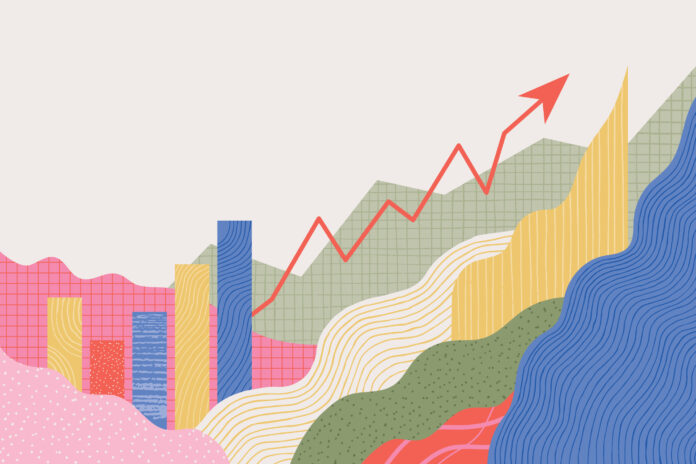
Time-series analysis is a powerful tool for businesses looking to gain insights into their data that evolve over time. In a dynamic, fast-paced business environment, understanding temporal patterns, trends, and fluctuations is critical for making informed decisions, predicting future outcomes, and optimizing operations.
What is Time-Series Analysis?
Time-series analysis takes a close look at data collected over time, where the information is gathered at regular intervals. In essence, this method provides a chronological view of the data, which is crucial for uncovering meaningful insights, patterns, trends, and relationships that can impact decision-making.
Time-series analysis serves as a fundamental tool for a wide range of applications, including finance, economics, epidemiology, meteorology, and more. Its utility extends to any domain where understanding how data evolves over time is essential.
This analytical approach enables professionals to explore the underlying structure of time-based data, make predictions, and extract actionable insights. By leveraging time-series analysis, individuals can forecast future values, identify the effects of specific interventions, and understand the causal relationships between variables over time.
5 Applications of Time-Series Analysis
Time-series analysis applies itself across a wide range of industries, contributing to better decision-making and resource allocation in impactful industries.
Here are 5 key areas where it’s commonly used:
1. Financial Forecasting
In the finance sector, time-series analysis helps in predicting stock prices, currency exchange rates, and economic indicators. It enables investors, traders, and financial institutions to make informed investment decisions.
2. Demand Forecasting
Manufacturing and retail businesses use time-series analysis to forecast product demand accurately. This ensures that inventory levels are optimized, reducing excess or insufficient stock.
3. Energy Consumption
Time-series analysis helps energy companies analyze consumption patterns, optimize energy production, and improve load management, resulting in cost savings and reduced environmental impact.
4. Epidemiology
In healthcare, time-series analysis is employed for tracking disease outbreaks, monitoring patient data, and predicting health trends, such as the spread of epidemics.
5. Weather Forecasting
Meteorologists use time-series analysis to predict weather conditions based on historical data, enabling them to issue accurate forecasts and warnings.
Common Time-Series Analysis Techniques
When delving into time-series analysis, it’s essential to understand the variety of techniques at your disposal. These techniques are chosen based on the characteristics of the data and the specific objectives you aim to achieve. Here, we’ll take a closer look at some of the common methods used in time-series analysis:
Moving Averages
Moving averages are a fundamental tool in time-series analysis. They are especially useful for identifying long-term patterns and trends within a dataset. This method involves calculating an average of data points over a defined window or time period. The resulting moving average provides a smoothed representation of the data, which helps filter out short-term fluctuations and noise. This, in turn, highlights the underlying trends.
Moving averages can come in various forms, such as simple moving averages (SMA), weighted moving averages, and exponential moving averages (EMA). The choice of which moving average to use depends on the specific characteristics of the data and the objectives of the analysis.
For example, if you are studying monthly sales data over several years, calculating a 12-month moving average can help identify yearly trends while smoothing out the monthly variations.
Exponential Smoothing
Exponential smoothing is another valuable technique for time-series analysis, particularly when dealing with short-term forecasting. This method assigns different weights to recent data points, giving more significance to the most recent observations. As the name suggests, the weighting is exponential, with recent data points contributing more to the forecast than older ones.
Exponential smoothing is well-suited for time series with seasonality, trend, and random noise. The method helps capture the latest patterns and changes in data while reducing the impact of historical data. In this way, it can provide more accurate forecasts for the immediate future.
For example, if you are managing inventory for an e-commerce business and need to predict the demand for a specific product for the next few weeks, exponential smoothing can help account for recent changes in demand patterns.
Autoregressive Integrated Moving Average (ARIMA)
The Autoregressive Integrated Moving Average (ARIMA) model is one of the most widely used techniques for time-series forecasting. It is particularly beneficial for datasets with trend, seasonality, and random fluctuations. ARIMA models incorporate parameters to address these characteristics systematically.
- Autoregressive (AR) terms consider the relationship between a data point and previous data points. They account for autocorrelation, meaning that the current value depends on past values.
- Integrated (I) denotes the number of differences needed to make the data stationary, i.e., to remove any trend. Data is considered stationary when its statistical properties, such as mean and variance, do not change over time.
- Moving Average (MA) terms consider the relationship between a data point and previous error terms (residuals). They help account for random noise or variability.
ARIMA models allow for a comprehensive understanding of time-series data by modeling the data’s history and identifying patterns and trends, even in the presence of randomness.
For instance, if you work for an airline and need to predict future ticket sales, an ARIMA model can handle the seasonality (e.g., increased sales during the holiday season), trends (e.g., long-term growth), and random variations that influence ticket sales.
Seasonal Decomposition
Seasonal decomposition is a method that breaks down a time series into its constituent components: seasonal, trend, and irregular. It provides insights into how these components behave over time.
- Seasonal Component: This component represents recurring patterns that occur at regular intervals. For example, in retail, it could capture the increase in sales every holiday season.
- Trend Component: The trend component shows the long-term direction of the data, indicating whether it’s increasing, decreasing, or remaining relatively stable over time.
- Irregular Component: The irregular component accounts for the unexplained fluctuations or noise in the data.
Seasonal decomposition helps you understand the fundamental components of your time series, making it easier to make sense of the data’s behavior and identify actionable insights. It’s particularly beneficial for identifying cyclical patterns and understanding how the past can inform future expectations.
For example, if you’re managing a hotel chain and want to analyze historical occupancy rates, seasonal decomposition can help identify patterns related to holidays, seasons, and other recurring factors.
What is Temporal Business Intelligence?
Temporal Business Intelligence, often referred to as TBI, is a specialized field within business intelligence that focuses on analyzing data over time. It involves the collection, processing, and interpretation of time-based data points to extract meaningful insights and patterns. TBI enables organizations to understand how specific metrics or variables change over time, providing valuable historical context for decision-making.
Temporal Business Intelligence is particularly well-suited for scenarios where time is a critical dimension, such as financial analysis, sales forecasting, and trend analysis. It leverages techniques like time-series analysis to uncover hidden trends, seasonality, and cyclical patterns in data.
5 Benefits of Temporal Business Intelligence
Businesses can derive several advantages from incorporating time-series analysis into their decision-making processes:
1. Improved Forecasting
Time-series analysis significantly enhances the accuracy of forecasts. This improvement in predictive accuracy equips businesses with the ability to plan more effectively, reduce risks, and allocate resources with greater precision. Whether it’s predicting sales trends, demand for specific products, or even the impact of external factors, a deeper understanding of time-based data empowers organizations to prepare for the future with confidence.
2. Identifying Trends and Anomalies
Temporal business intelligence allows organizations to identify trends and patterns within their data. This includes the detection of seasonal variations and the timely recognition of anomalies or irregularities. Being aware of these trends and outliers enables businesses to take prompt and well-informed action, optimizing their operations. For instance, a retailer can proactively adjust inventory levels based on historical sales trends to meet customer demand.
3. Data-Driven Decision Making
Leveraging historical data for analysis promotes data-driven decision making. Businesses can make informed choices based on quantitative insights rather than relying on intuition or guesswork. This transition to data-driven decision making not only minimizes the risks associated with subjective judgments but also increases the chances of success in various strategic initiatives.
4. Resource Optimization
Time-series analysis can be invaluable in optimizing resource allocation. Organizations can efficiently manage resources, such as personnel, inventory, energy, and more. This optimization reduces waste and cuts down on operational costs. For instance, energy consumption can be reduced during non-peak hours based on historical usage patterns, leading to substantial cost savings.
5. Competitive Advantage
Embracing temporal business intelligence provides companies with a competitive edge. It enables businesses to adapt swiftly to changing market conditions and evolving customer preferences. By staying attuned to the latest trends and customer behaviors, organizations can position themselves effectively, ensuring that they remain competitive and responsive to market shifts.
Challenges in Time-Series Analysis
While time-series analysis offers substantial benefits, it also presents challenges, including data quality issues, data gaps, and the need for sophisticated modeling techniques. Additionally, interpreting results requires a sound understanding of statistical concepts.
Empower Your Business with Time-Series Analysis
In an era where data is hailed as the lifeblood of business, the importance of time-series analysis cannot be overstated. By embracing this powerful technique, organizations gain a competitive advantage, making informed decisions, optimizing resources, and foreseeing future trends.
Temporal Business Intelligence is more than just a tool; it’s a strategic approach that helps businesses navigate the complexities of a rapidly evolving landscape. So, consider integrating time-series analysis into your decision-making processes to unlock the full potential of your historical data.
As your business harnesses the power of time-series data, remember that the past is not just history; it’s a blueprint for a brighter future.