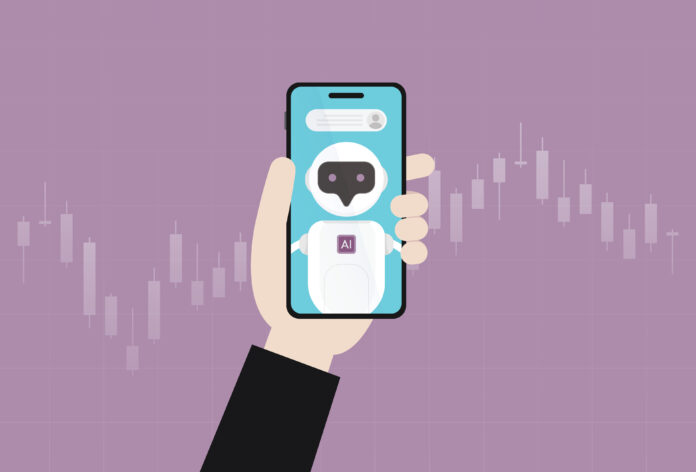
The use of artificial intelligence (AI) in credit scoring has become increasingly prevalent in the financial industry. However, concerns about the opacity of AI models have raised questions about fairness, accountability, and the potential for bias. In this article, we explore the role of explainable AI (XAI) in credit scoring, emphasizing the importance of building transparent models to ensure fair lending practices.
The Rise of AI in Credit Scoring
Traditional credit scoring methods have long been the cornerstone of assessing an individual’s creditworthiness. However, the advent of AI has introduced advanced analytical techniques that promise improved accuracy and efficiency in evaluating credit risk. Machine learning algorithms, particularly deep neural networks, have demonstrated the ability to analyze vast datasets and extract intricate patterns that may elude traditional scoring models.
The Challenge of Opacity in AI Models
While AI models offer enhanced predictive capabilities, their complexity often results in a lack of transparency. This opacity can lead to skepticism and apprehension, especially in sensitive areas such as credit scoring. Stakeholders, including consumers, regulatory bodies, and financial institutions, demand a clear understanding of how credit decisions are made to ensure fairness and prevent discriminatory practices.
Explaining AI Decisions through Explainable AI
Explainable AI (XAI) addresses the challenge of opacity by providing insights into the decision-making process of AI models. In the context of credit scoring, XAI aims to make the logic and factors influencing credit decisions more understandable and interpretable. This transparency is crucial for fostering trust among stakeholders and ensuring that credit scoring models adhere to fair lending practices.
Feature Importance Analysis
XAI techniques enable the identification of key features that contribute to credit decisions. By analyzing feature importance, stakeholders can gain insights into which factors significantly impact an individual’s credit score. This transparency allows for the identification and mitigation of any potential biases in the model.
Model-agnostic Approaches
Model-agnostic XAI methods can be applied to a variety of machine learning models, providing a standardized approach to transparency. Techniques such as LIME (Local Interpretable Model-agnostic Explanations) and SHAP (SHapley Additive exPlanations) offer post-hoc explanations, allowing stakeholders to interpret the decisions of complex AI models.
Human-Readable Explanations
XAI goes beyond technical insights by providing human-readable explanations of AI decisions. This is crucial for ensuring that individuals, including those without a technical background, can understand the factors influencing their credit scores. Human-readable explanations contribute to increased transparency and accountability.
Fair Lending Practices and Regulatory Compliance
Explainable AI aligns credit scoring practices with fair lending principles and regulatory requirements. Transparency in AI models ensures that credit decisions are not influenced by discriminatory factors such as race, gender, or socioeconomic status. This is vital for upholding fair lending practices and complying with regulations such as the Equal Credit Opportunity Act (ECOA) and the Fair Credit Reporting Act (FCRA).
Striking a Balance: Accuracy vs. Interpretability
One challenge in implementing XAI in credit scoring is striking the right balance between accuracy and interpretability. While complex models may offer superior predictive performance, ensuring that these models are interpretable remains crucial. Striking this balance is essential to build models that are both reliable and transparent.
The Future of Credit Scoring with Explainable AI
The integration of XAI into credit scoring models marks a significant step toward fostering trust, fairness, and accountability. As technology continues to evolve, innovations in XAI will likely play a pivotal role in shaping the future of credit scoring. Financial institutions that prioritize transparency and fairness in their credit decisions will not only comply with regulatory requirements but also build stronger relationships with consumers.
XAI Makes Fair Lending More Fair
Explainable AI is a pivotal component in revolutionizing credit scoring practices, ensuring transparency, fairness, and compliance with regulatory standards. As financial institutions increasingly adopt AI in their decision-making processes, the role of XAI becomes paramount in building models that are not only accurate but also interpretable. The journey toward fair lending practices requires a commitment to leveraging XAI to unravel the complexities of AI models, empowering stakeholders with the understanding they need to trust and embrace the transformative potential of AI in credit scoring.